Tables of Content
1. Introduction
Medical billing is only one of the many industries that frequently use the potent data analysis approach known as clustering. By combining related data points based on predetermined criteria, the clustering process enables the detection and analysis of patterns and insights.
Clustering is a technique used in medical billing to group medical claims according to various criteria, such as the kind of service rendered, diagnosis code, or insurance provider. Medical billing specialists can use this technique to analyse trends in their billing data, spot potential fraud or billing problems and enhance revenue cycle management.
In this article, we will look at the main ideas behind clustering in medical billing, and its advantages and practical applications.
2. Clustering in Medical Billing: Advantages
Medical billing is a difficult and dynamic procedure that needs a lot of data to be carefully managed. Clustering in medical billing has many advantages, including:
Detecting Potential Billing or Fraud Issues
Medical billing fraud is a serious problem that can cost healthcare organizations a lot of money. Data on medical claims can be clustered to find trends that might point to possible fraud or billing mistakes. For instance, clustering can group claims submitted for the same diagnostic code or by the same provider together and point up potential differences that call for an additional examination.
Examining Billing Data Trends
Professionals that handle medical billing can use clustering to examine trends in their billing data and learn more about the revenue cycle management process. Healthcare organizations can, for instance, determine which services are most commonly utilised, the typical reimbursement rate for each service, and the revenue produced by each service by grouping claims based on the type of service supplied. To enhance revenue cycle management and boost financial performance, use this information.
Enhancing the Submission and Processing of Claims
Clustering can also be utilized to speed up the procedure for filing and handling claims. Healthcare organizations can determine which insurers have the greatest claim denial rates or demand more proof by grouping claims according to the insurance carrier. Utilizing this information helps lower the possibility of claim denials and streamlines the claim filing and processing procedures.
Key Clustering Concepts in Medical Billing
It is crucial to comprehend a few fundamental ideas to efficiently employ clustering in medical billing.
Clustering Conditions
The properties or traits that are utilized to combine data from medical claims are called clustering parameters. The diagnosis code, provider ID, or insurance company are just a few examples of the various components of the medical claims data that can be used to base the clustering parameters. The analysis goal and the kind of insights the medical billing specialist hope to obtain will determine the clustering parameters to use.
Clustering Method
Based on the clustering parameters, medical claims data are grouped together using a clustering algorithm. K-means, hierarchical clustering, and DBSCAN are just a few of the many clustering methods available. The size and complexity of the medical claims data collection as well as the level of precision that is sought in the analysis all influence the algorithm that is used.
Cluster Analysis
The process of evaluating the quality of a clustering result is called a cluster evaluation. Different metrics, such as the within-cluster sum of squares, the silhouette score, or the Davies-Bouldin index, might be used to base the evaluation. The cluster evaluation procedure is essential for ensuring that the clustering results are precise and pertinent to the analysis goal.
3. Clustering in Medical Billing: How to Use It
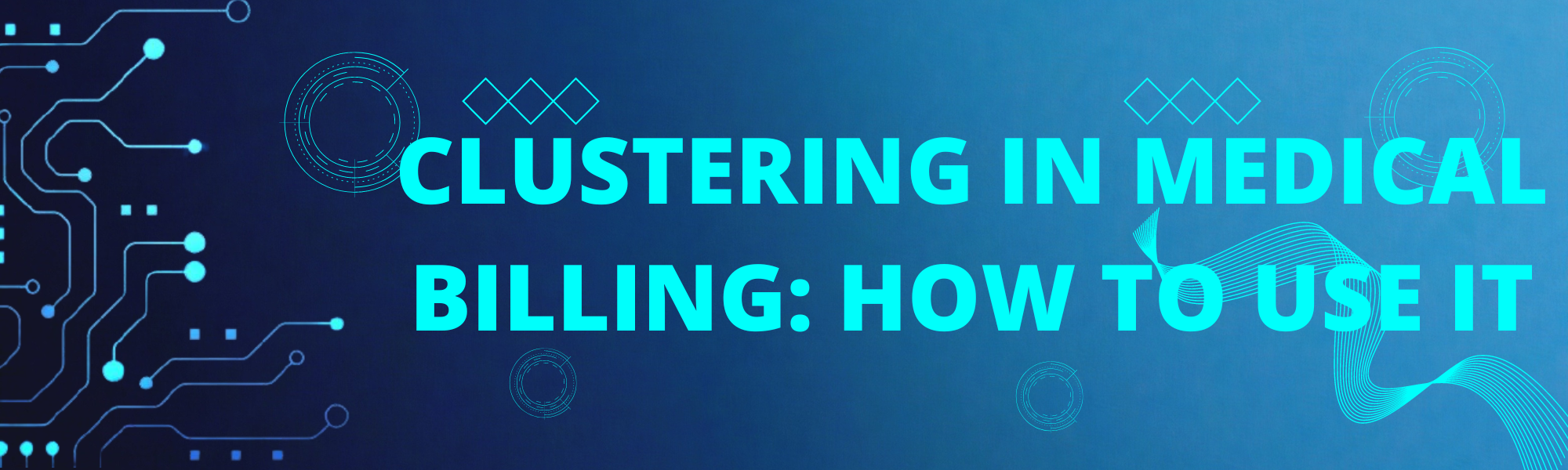
Observe these measures to efficiently use clustering in medical billing:
Define the goal in Step 1
The analysis goal must be specified before clustering may be used in medical billing. The goal may be to find potential fraud or billing mistakes, examine billing data trends, or enhance the claim submission and processing procedures.
Choose the Clustering Parameters in Step 2
The clustering parameters are selected after the objective has been established. The analysis goal and the kinds of insights the medical billing specialist hope to glean should guide the selection of clustering parameters.
Choose the Clustering Algorithm in Step 3
Selecting the clustering algorithm comes after picking the clustering parameters. There are numerous clustering methods available, each with its own advantages and disadvantages, as was previously noted. The size, complexity, and desired accuracy in the analysis should all be considered while selecting an algorithm.
Prepare the data in Step 4
The preparation of medical claims data is necessary before performing the clustering analysis. This entails preparing the data for clustering analysis by cleaning it, eliminating any duplicates or pointless material, and putting it into the appropriate format.
Perform the Clustering Analysis in Step 5
The clustering analysis can be carried out using the chosen technique and clustering parameters once the data has been prepared. Based on the chosen parameters, the clustering analysis results will divide the data from medical claims into clusters.
The evaluation of the Clustering Results in Step 6
It is crucial to assess the quality of the clustering findings using the right metrics after performing the clustering analysis. This will guaranty that the findings are precise and pertinent to the analysis goal.
Analyse the Findings in Step 7.
The final stage is to analyse the clustering analysis findings and make judgments based on the knowledge gathered. Through the analysis of billing data trends and the identification of potential fraud and billing problems, this information can help streamline the claim submission and processing procedures.
4. Conclusion
A strong data analysis method called clustering can provide important information about medical billing data. By combining related data points based on predetermined criteria, the clustering process enables the detection and analysis of patterns and insights.
Healthcare organizations can discover potential fraud or billing issues, examine trends in billing data, and enhance the claim submission and processing process by using clustering in medical billing.
It is crucial to comprehend the fundamental ideas behind clustering parameters, clustering algorithms, and cluster evaluation to use clustering in medical billing efficiently. Medical billing professionals can gain important insights and enhance the overall revenue cycle management process by using a structured approach that includes defining the objective, selecting the clustering parameters, selecting the clustering algorithm, preparing the data, performing the clustering analysis, evaluating the results, and interpreting the results.