1. Introduction
Speech recognition, natural language processing, audio categorization, voice assistants, and other fields all rely heavily on audio annotation. Researchers and developers may teach machine learning models to precisely read and comprehend spoken language or audio material by adding relevant annotations to audio data.
One of the most common types of audio annotation is transcription. It entails converting verbatim text from audio files of spoken speech. Keyword searches, content indexing, and spoken content analysis are all made possible via transcriptions. Furthermore, timestamping is utilized to pinpoint the exact moments when particular phrases or events take place in the audio, enabling accurate analysis and referencing.
Another crucial component of audio annotation is speaker recognition. It entails giving each speaker in a discussion or audio recording its own labels. It is feasible to distinguish between individual speakers, keep track of their contributions, and conduct speaker-specific analysis by associating speakers with distinctive identifiers, such as speaker numbers or names.
Sentiment analysis is a useful annotation method for deciphering audio’s subjective or emotional content. Sentiment analysis is a technique that aids in the analysis of customer feedback, voice-based surveys, and audio recordings on social media by recognizing and categorizing the emotions represented in the audio, such as happiness, sorrow, anger, or neutrality.
Additionally, labelling certain audio occurrences or classes may be included in audio annotation. For instance, audio data may be tagged with tags indicating the presence of particular sounds, such as dogs barking, automobile horns, or musical instruments, in audio classification tasks. This makes it possible to create applications for audio-based surveillance, environment monitoring, and sound recognition systems.
In conclusion, audio annotation is an important step that improves the usability and comprehension of audio data. It enables audio material to be effectively understood and analyzed by machine learning models, supporting a variety of applications from sentiment analysis to audio categorization and voice recognition. Audio data may be made more available, searchable, and useful for a variety of companies and research sectors by adding metadata and annotations.
Any audio production should have annotations. It is a strong tool with a wide range of further applications. It can enhance the precision of voice recognition systems, offer more accurate translations, and aid in producing more realistic synthetic speech, among many other advantages.
However, it also has certain drawbacks, such as the requirement for excellent audio recordings and the possibility of incorrect annotation.
2. Different typees of Audio Annotation
When it comes to voice recognition, natural language processing, audio classification, and sentiment analysis, audio annotations are crucial for maximising the potential of audio data. Audio annotations enable machine learning models and applications to recognise and interpret audio material properly by labelling or tagging audio files with descriptive information.
We shall examine several audio annotations that improve the accessibility and usefulness of audio data in this post. These annotations include a wide range of methodologies and metadata, enabling thorough examination, indexing, and information extraction from audio recordings.
First, we explore the field of transcription, which entails turning spoken words in audio recordings into written text. By facilitating keyword search, content indexing, and linguistic analysis, transcriptions serve as the framework for comprehending and analysing spoken information. We discuss the value of proper transcriptions and the difficulties associated with turning audio into text.
The process of labelling various speakers in a conversation or audio recording is known as speaker identification, and it is a crucial component of audio annotation. It is feasible to distinguish between individual speakers, keep track of their contributions, and conduct speaker-specific analysis by associating speakers with distinctive identifiers, such as speaker numbers or names. We go through the importance of speaker identification in contexts including forensic investigations, voice assistants, and contact centre analytics.
Then we go to sentiment analysis, which is concerned with finding and labelling emotions represented in audio data. Annotations for sentiment analysis offer information on the emotional or subjective content of audio, such as happiness, sorrow, rage, or neutrality. We look at how sentiment analysis may be used in customer feedback analysis, social media monitoring, and voice-based surveys.
In addition, we look for annotations relating to certain audio occurrences or classes. Labelling audio data with tags identifying the existence of certain sounds, such as animal sounds, musical instruments, or ambient noises, falls under this category. These annotations make it possible to create sound recognition systems, soundscape analysis software, and audio-based surveillance applications.
We emphasise the importance of audio annotations in numerous sectors and research domains throughout our examination of them. Audio annotations are essential for maximising the potential of audio data, from enhancing speech recognition algorithms to enabling advanced audio analysis. We are better able to appreciate the potential of audio in the data-driven world of today by comprehending the many sorts of audio annotations and their uses.
Audio annotations encompass a variety of techniques that add descriptive information to audio data, making it more understandable and useful for machine learning applications. Transcription involves converting spoken words in audio files into written text, enabling keyword search, indexing, and linguistic analysis.
Speaker identification labels different speakers within an audio recording, allowing for speaker-specific analysis and tracking contributions. Sentiment analysis focuses on identifying and labeling emotions expressed in audio, facilitating customer feedback analysis and social media monitoring.
Additionally, annotations can be applied to specific audio events or classes, such as tagging animal sounds or musical instruments, enabling sound recognition and environmental analysis. These types of audio annotations enhance the usability and interpretability of audio data, opening possibilities for improved speech recognition, audio classification, and sentiment analysis in various industries and research fields.
3. Types in Detail
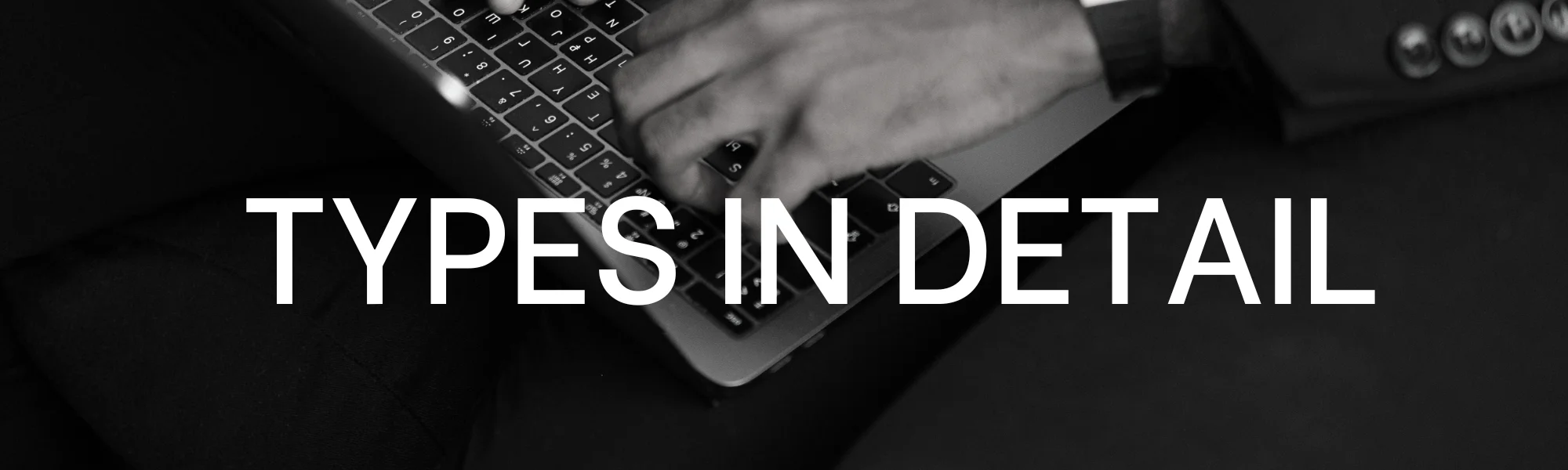
ASR, commonly referred to as voice-to-text conversion, is a transformational technology that turns spoken language into written text. It is essential to several fields, including voice assistants, closed captioning, language translation, transcription services, and more. Speech-to-text transcription makes it easier to access, retrieve information from, and analyses audio recordings by allowing spoken words to be converted into written words.
Transcribing spoken words into text includes multiple steps. The audio data is first recorded from a source, such as a phone call or video, using a microphone, or it may be manually recorded. The acoustic data from the audio are then transformed into text representations utilising sophisticated algorithms and machine learning models. Pre-processing, acoustic modelling, and language modelling make up the three primary phases in this procedure.
The audio signal goes through a number of changes during pre-processing to improve its quality and get it ready for analysis. Identifying distinct speech segments, this may involve noise reduction, normalization, filtering, and segmentation. These preprocessing methods lessen background noise and other interferences, which helps to increase the accuracy of the transcription output.
Acoustic modelling is the following step, when audio characteristics are mapped to phonetic units or speech sounds using a machine learning model that has been trained. Large volumes of labelled audio data with known related transcriptions are used to do this. Deep neural networks and other machine learning algorithms are taught to understand the correspondence between audio data and the associated phonetic units. As a result, the model can identify and distinguish between various speech sounds in the audio.
The last stage of speech-to-text transcription is language modelling. It focuses on adding linguistic context and enhancing the output transcription's overall correctness. When given audio data, language models use statistical methods and linguistic expertise to forecast the most likely word order. The transcription system may produce more precise and coherent transcriptions by taking word probabilities, grammatical rules, and contextual information into account.
Systems for automatic speech recognition have come a long way in recent years, yet there are still problems. The accuracy of the transcriptions can be impacted by changes in speech patterns, accents, background noise, and speech disfluencies.
Additionally, speech that uses colloquial language or terminology peculiar to a certain domain might be challenging for voice recognition algorithms. The performance of speech-to-text transcription systems is being enhanced through ongoing research, machine learning algorithm developments, and data gathering methods.
Speech-to-text transcription has a plethora of different uses. For example, transcription services rely on precise speech-to-text conversion to provide written recordings of conferences, meetings, and interviews. This makes it simple to archive, reference, and search the spoken material. Voice assistants, like Apple Siri, Google Assistant, and Amazon Alexa, employ voice recognition to comprehend user requests, carry out tasks, and deliver information.
Additionally, transcription from speech to text is essential for accessibility services. Individuals with hearing disabilities can access and understand the audio content of videos by offering closed captions or subtitles. The transcribed text may be simply translated into other languages, facilitating multilingual communication and information exchange. It also makes language translation easier.
An innovative technique that transforms spoken words into written text is voice to text transcription. From voice assistants and transcription services to closed captioning and language translation, it has transformed a variety of industries and applications.
Speech-to-text transcription systems offer accessibility, information retrieval, and analysis of audio content using a mix of pre-processing, acoustic modelling, and language modelling approaches. This makes spoken language more useable and accessible in the digital era.
Music is annotated and categorized into several genres or classes based on its musical features in a process known as musical classification. It is essential to several fields, including music recommendation systems, music streaming services, music analysis, and content management. It is feasible to improve music discovery, personalization, and comprehension of musical patterns and trends by correctly categorizing music.
The main processes in the categorization of music are to extract pertinent characteristics from the audio input and train machine learning models to recognize and distinguish between various genres or classes. Data gathering, feature extraction, model training, and classification are some of these phases.
The basis for classifying music is data collecting. Audio samples from various genres or classes are combined to create a broad and representative collection. For machine learning models, this dataset acts as the training set. When the audio samples are labelled with the correct genres or classes, the models may learn the patterns and traits that belong to each category.
A critical stage in musical categorization is feature extraction. From the audio signals, several audio characteristics are retrieved that reflect various facets of the music, including rhythm, pitch, timbre, and spectral content.
Mel-frequency cepstral coefficients (MFCCs), spectral features, rhythmic features, and tonal features are examples of frequently utilized features. These variables serve as inputs to the machine learning models and capture the distinctive qualities of the music.
Machine learning models are taught to categories the music into several genres or classes once the characteristics are retrieved. Support vector machines (SVM), random forests, or deep neural networks are examples of supervised learning techniques that are frequently employed for this job.
The audio attributes are used as the input and the genre or class labels are used as the output in the labelled dataset on which the models are trained. Based on the retrieved characteristics, the models train themselves to recognize and distinguish between various genres or classes.
The models may be used to categories brand-new, unheard music once they have been taught. The trained models receive the audio attributes that were derived from the new music and forecast the most likely genre or class label for the input music. The categorization result may be applied to a variety of tasks, including managing music collections, developing individualized music
Handling ambiguity, comprehending context, and coping with out-of-vocabulary words or phrases are difficulties in natural language utterance categorization. Given the complexity of spoken language, statements may have several different meanings or subtleties.
It can sometimes be difficult to distinguish between synonyms and homonyms and grasp the context. The performance of utterance classification systems is being enhanced by ongoing research and developments in machine learning, including the use of contextual language models like BERT.
The categorization of utterances in natural language has changed how we communicate with text-based technologies. It makes it possible for chatbots and virtual assistants to comprehend customer inquiries and offer pertinent and correct answers. Additionally, it supports sentiment analysis, ticket routing for customer service, and data analysis of substantial text corpora.
Natural language utterance categorization is advancing and helping to create intelligent, context-aware language processing systems by utilising machine learning algorithms and sophisticated feature extraction methods.
Labelling speech is the practice of labelling or classifying spoken language segments inside audio recordings in accordance with predetermined standards. It is an important stage in many applications, such as voice recognition, speaker identification, sentiment analysis, and transcription services.
These algorithms may increase accuracy, comprehend various speakers, examine emotional content, and provide meaningful transcriptions by precisely labelling voice segments. The labelling of speech is a multi-step process that comprises data collection, segmentation, labelling, and validation.
The initial stage in labelling speech is data collecting. The audio recordings are compiled into a broad and representative dataset that includes speech fragments from various speakers, settings, or subjects. To provide robustness in the labelling process, the dataset should encompass a variety of language variances, accents, and background noise levels.
The technique of segmentation involves breaking down audio recordings into more manageable chunks, such phrases or individual utterances. For the purpose of separating out distinct speech segments and getting them ready for labelling, this step is crucial.
Segmentation can be done automatically using methods based on energy thresholds or quiet detection, or manually using human annotators to mark the borders between speech segments.
Depending on the size and complexity of the work, the labelling process can be carried out manually by human annotators or using automated systems. In manual annotation, trained individuals listen to the audio and assign labels in accordance with given instructions. To anticipate labels for fresh speech segments, automated systems may use machine learning algorithms trained on labelled data.
In order to guarantee the precision and dependability of the labelled speech segments, validation is a crucial step. This entails checking the accuracy of the annotations and correcting any errors or inconsistencies.
Multiple annotators can independently identify the same speech segments, and validation can be done by comparing the labels to see whether they agree. As an additional quality assurance measure, annotators are trained, regularly monitored, and given feedback.
Dealing with accents, speech changes, and background noise in the audio are difficulties in labelling speech. The achievement of consistent and accurate labelling might be complicated by differences in speech patterns, pronunciation, and language usage. The accuracy of transcriptions or speaker identification can also be affected by ambient noise or poor audio recordings.
Despite these difficulties, precise speech labelling is essential for creating trustworthy speech recognition systems, comprehending speaker traits, assessing emotional content, and producing transcriptions. The labelling process aids in the development of several applications that depend on voice data, either manually or automatically.
Labelling speech, then, is the process of marking or classifying spoken language fragments inside audio recordings. Applications like voice recognition, speaker identification, sentiment analysis, and transcription services all heavily rely on it. The method entails data collection, segmentation, labelling, and validation, allowing for precise speech data analysis, comprehension, and processing.
The technique of classifying and annotating spoken or written language utterances into distinct classes or categories based on their semantic meaning or purpose is known as natural language utterance categorization. It is essential to many applications, including sentiment analysis, chatbots, virtual assistants, and customer care systems.
These systems are able to recognize natural language utterances, comprehend user requests, deliver pertinent replies, and glean insightful information from text data.Data gathering, feature extraction, model training, and classification are all processes in the process of classifying natural language utterances.
The first stage in creating a system for classifying natural language utterances is data collecting. We collect instances of various utterances that have been labelled in order to create a varied and representative dataset. These statements might be made in the form of user inquiries, service requests, or social media updates. The related categories or intents are meticulously marked in the dataset and used as the basis for training the classification model.
A crucial stage in classifying natural language utterances is feature extraction. The raw text data is transformed using a variety of methods into numerical representations that capture the semantic content of the utterances.
Bag-of-words, word embeddings (like word2vec or GloVe), and more sophisticated algorithms like BERT (Bidirectional Encoder Representations from Transformers) are some of the frequently used feature extraction techniques. These characteristics provide a foundation for categorization by capturing the contextual and semantic information of the utterances.
Machine learning models are taught to categorise the natural language utterances into several categories or intentions once the characteristics are retrieved. Support vector machines (SVM), random forests, or deep neural networks are examples of supervised learning techniques that are frequently employed for this job.
The extracted features from the labelled dataset are used as input for the models during training, and the output is either the category or intent labels. The models learn the patterns and connections between the characteristics and the related categories or intents throughout the training phase.
Handling ambiguity, comprehending context, and coping with out-of-vocabulary words or phrases are difficulties in natural language utterance categorization. Given the complexity of spoken language, statements may have several different meanings or subtleties.
It can sometimes be difficult to distinguish between synonyms and homonyms and grasp the context. The performance of utterance classification systems is being enhanced by ongoing research and developments in machine learning, including the use of contextual language models like BERT.
The categorization of utterances in natural language has changed how we communicate with text-based technologies. It makes it possible for chatbots and virtual assistants to comprehend customer inquiries and offer pertinent and correct answers.
Additionally, it supports sentiment analysis, ticket routing for customer service, and data analysis of substantial text corpora. Natural language utterance categorization is advancing and helping to create intelligent, context-aware language processing systems by utilising machine learning algorithms and sophisticated feature extraction methods.
1. Automatic speech recognition
2. Musical classification
3. Labelling speech
4. Natural Language utterance
4. Conclusion
In conclusion, we have explored the world of audio annotations, encompassing various techniques and applications. We began by understanding the importance of audio annotations in enhancing the usability and interpretability of audio data. Transcription enables spoken words to be converted into written text, opening doors for keyword search, linguistic analysis, and indexing.
Speaker identification allows for the identification and tracking of different speakers within audio recordings, enabling speaker-specific analysis. Sentiment analysis focuses on identifying emotions expressed in audio, facilitating customer feedback analysis and social media monitoring. Annotations can also be applied to specific audio events or classes, such as animal sounds or musical instruments, enabling sound recognition and environmental analysis.
We then delved into specific domains of audio annotation. Speech-to-text transcription, also known as automatic speech recognition (ASR), involves converting spoken language into written text. This technology has revolutionized transcription services, voice assistants, language translation, and closed captioning, enabling accessibility and information retrieval from audio content.
Musical classification, on the other hand, focuses on annotating and categorizing music into different genres or classes based on its musical characteristics. This process enhances music recommendation systems, music analysis, and content organization, providing personalized music recommendations and insights into musical patterns and trends.
Lastly, we explored natural language utterance classification, which involves categorizing and annotating spoken or written language utterances based on their semantic meaning or intent. This technology powers chatbots, virtual assistants, customer support systems, and sentiment analysis, enabling understanding of user queries, appropriate responses, and extraction of valuable insights from text data.
Overall, audio annotations play a pivotal role in unlocking the potential of audio and textual data across various industries and research fields. From speech-to-text transcription to musical classification and natural language utterance classification, these techniques improve accessibility, accuracy, and understanding of audio content. As technology advances and new methodologies emerge, audio annotations continue to evolve, driving innovation and enabling new possibilities in the world of audio data analysis.
Medreck Company has emerged as a leading player in the field of audio annotations, showcasing remarkable success in various aspects of the industry. With their cutting-edge technologies and expertise, Medreck has revolutionized the transcription services market by providing accurate and efficient speech-to-text conversion.
Their advanced algorithms and machine learning models have enabled them to achieve high levels of accuracy in transcribing spoken language into written text, making audio content easily searchable and accessible. Moreover, Medreck's commitment to continuous improvement and adaptation to new advancements in the field has allowed them to stay ahead of the competition.
Their robust systems for musical classification and natural language utterance classification have further cemented their position as industry leaders, enabling personalized music recommendations, intelligent chatbots, and sentiment analysis. Through their dedication to excellence, Medreck Company has not only contributed to the advancement of audio annotations but has also empowered businesses and individuals to unlock the full potential of audio and textual data.